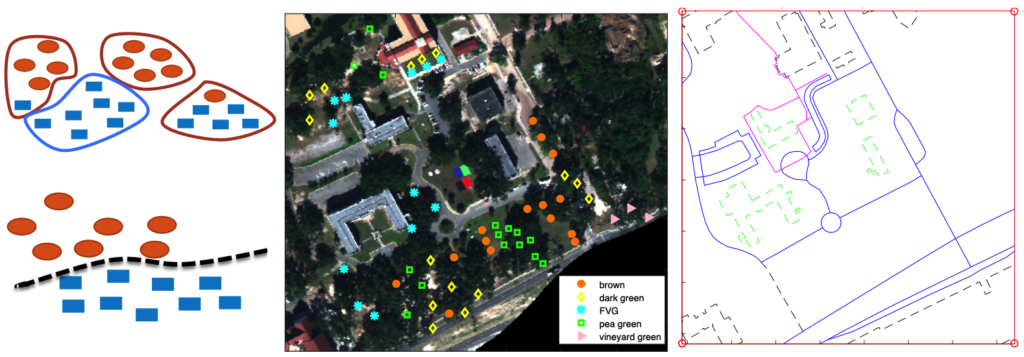
In sensor fusion, the aim is to combine the outputs of several algorithms to boost overall performance in applications such as target detection and scene understanding. Previous supervised fusion algorithms often require accurate and precise training labels for each pixel/data point. However, accurate labels are difficult, expensive, or simply impossible to obtain for real, large-scale datasets. A series of novel classification and regression fusion methods were developed to learn from ambiguously and imprecisely labeled training data. Notably:
MICI — for two-class classifier fusion and regression with imprecise labels.
MIMRF — for multi-resolution, multi-modal sensor fusion with label uncertainty.
- MIMRF with Binary Fuzzy Measure
Associated Publications
- Efficient Multi-Resolution Fusion for Remote Sensing Data with Label Uncertainty, International Geoscience and Remote Sensing Symposium (IGARSS), July 2023.
- [Book Chapter] Addressing the Inevitable Imprecision: Multiple Instance Learning for Hyperspectral Image Analysis, in Hyperspectral Image Analysis, pp. 141-185, Springer, Cham, 2020.
- Multiresolution Multimodal Sensor Fusion For Remote Sensing Data With Label Uncertainty, IEEE Transactions on Geoscience and Remote Sensing, 2019.
- Multiple Instance Choquet Integral Classifier Fusion and Regression for Remote Sensing Applications, IEEE Transactions on Geoscience and Remote Sensing, 2019.
- Multiple Instance Choquet Integral with Binary Fuzzy Measures for Remote Sensing Classifier Fusion with Imprecise Labels, IEEE Symposium Series on Computational Intelligence (SSCI), 2019.
- Multiple Instance Choquet Integral for Classifier Fusion, IEEE Congress on Evolutionary Computation (CEC), 2016.
- Multiple Instance Choquet Integral For Multi-Resolution Sensor Fusion, PhD dissertation, 2017.